Please note: This framework is a work-in-progress that has only been trialed a few times, and this post serves as an invitation to readers to comment—it is open to improvement!
Introduction
This post describes a methodology for the application of quantified collective intelligence (QCI) to decision-making in a policy context, leveraging the combined advantages of expert knowledge and calibrated human judgmental forecasting. The structured combination of these elements can buttress the process of decision-making and policy development, reducing bias and noise and increasing transparency and interpretability, thereby potentially leading to more robust and resilient plans and outcomes.
Components of the Quantified Collective Intelligence Framework
Laying the Groundwork: Structured Scenario Development
Facilitating the development of scenarios is the first step, since they will serve as a foundation for the decision-making process. The scenarios can be organized around any one of three potential pillars: Uncertainty-driven scenarios allow for versatile and flexible decision-making (utilizing a 2x2, or n x n matrix format), goal-driven scenarios are centered on a defined vision, acting as a beacon for future strategic moves, and risk-driven scenarios are tailored to anticipate and prepare for known risks, ensuring organizational resilience.
Uncertainty-driven Scenarios
Goal-driven Scenarios
Risk-driven Scenarios
Nature and Focus
Wide-ranging and exploratory, focusing on diverse outcomes stemming from a broad spectrum of uncertainties.
Highly strategic and focused on a specific end-goal, serve the purpose of detailing pathways toward a desired future.
Targeted to focus on known risks and the organization’s resilience against them.
Types of Uncertainty
Best for addressing complex and interdependent uncertainties that could lead to a variety of futures.
Best for situations with uncertainty about how to reach a desired and defined future state.
Best for situations with uncertainty around known risks and their potential impacts on the organization.
Process & Expertise
The process involves identifying key uncertainties, constructing a scenario matrix, and interpreting the outcomes. Requires both internal and external expertise for a broad perspective on uncertainties.
The process involves defining a clear future vision, backcasting from that future to present, and strategizing the path forward. Primarily requires internal expertise, with external insights for strategic approaches.
The process involves identifying key risks, understanding their potential impacts, and developing response strategies. Requires a combination of internal and external expertise for effective risk management.
Timeframes
Typically long-term, as the impact of uncertainties often unfolds over extended periods. However, this can be adjusted according to specific needs.
Can be used in both short and long term, but is particularly useful for long-term strategic planning where the organization has a specific goal or vision for the future.
Typically short to medium term, as the risks being focused on are usually imminent or visible in the near future.
Examples
A 2x2 matrix could be set up with the economy on one axis and the environment on the other. Four scenarios would then be generated: strong economy-stable environment, weak economy-stable environment, strong economy-volatile environment, and weak economy-volatile environment
A goal driven scenario might consist of a detailed vision an organization has already articulated. This could be in the form of an ambitious goal, such as the complete eradication of a disease, or the output of a vision-setting exercise in which a specific desired future state has been described.
Examples of known risks that can be formulated into scenarios include nuclear use, pandemics, and the use of AI deepfakes to influence and manipulate political processes.
Uncertainty-driven Scenarios: 2x2, n x n
These types of scenarios are designed to explore the potential impact of various uncertainties, usually across multiple dimensions, hence the use of the 2x2 or n x n matrices to represent the most important axes of uncertainty at hand. For example, a 2x2 matrix could be set up with the economy on one axis (ranging from strong to weak) and the environment on the other (ranging from stable to volatile). Four scenarios would then be generated: strong economy-stable environment, weak economy-stable environment, strong economy-volatile environment, and weak economy-volatile environment. The aim of this approach is to create a breadth of potential futures that cover a wide spectrum of possibilities, thereby preparing the organization or decision-maker for a diverse range of outcomes.
Goal-driven Scenarios: Move toward a clearly defined vision
Unlike uncertainty-driven scenarios, goal-driven scenarios start with a clear vision or end goal in mind. This approach backcasts from a desired future to the present, plotting a course that bridges the gap between the current reality and the envisioned future. Goal-driven scenarios make sense when the relevant stakeholders have already defined where they want the organization to go, and have some idea of the steps needed to get there. It’s beneficial in terms of building commitment and understanding of the goal across an organization, and is particularly useful for situations where there’s a high level of agreement about the preferred future, and the challenge lies in defining how to achieve it.
Risk-driven Scenarios: Ensure robustness against known risks
Risk-driven scenarios focus on ensuring robustness and resilience against known or identified risks. They model the impacts of specific risks and how they can be mitigated or managed. This type of scenario planning requires the identification of key risks, understanding their potential impacts, and then developing scenarios that test different response strategies. The primary objective is to stress-test strategies against these risks to ensure resilience. It provides an in-depth understanding of specific threats, assists in the preparation for potential disruptions, and allows for proactive risk management.
Quantitative Capture of Mental Models in Scenario Construction
A vital part of scenario construction lies in the understanding and translation of mental models into quantifiable entities. Mental models, representing individual or collective understanding and interpretation of how the world works, serve as the foundation upon which scenarios are built. These models help identify key variables, the relationships among them, and their potential future states, providing a basis for the narrative of the scenario. When these mental models are captured quantitatively, they become computationally tractable objects, lending themselves to precise and consistent analysis and supporting more accurate decision-making and future learning. The process of capturing mental models can be achieved through techniques such as Bayesian networks, system dynamics, or agent-based modeling, which can represent complex interdependencies and uncertainties.
By facilitating the capture of mental models into their quantified counterparts during scenario construction, we can integrate them into the QCI framework, allowing these models to evolve dynamically and be carried through each step of the overarching decision-making process.
Getting Leverage: Identifying Driving Indicators
The next stage involves Identifying Driving Indicators (DIs). These are factors that directly influence scenarios and are therefore crucial to our ability to track progress toward a given scenario or end state. A DI can be measurable or qualitative, as long as the group of experts can agree on its definition and value, allowing for its later integration into a resolution council process during the forecasting stage. Examples of quantitative DI’s might be economic growth, or the number of Covid deaths in a given year, whereas qualitative DI’s might be a leader’s reputation within the international community, or public confidence in institutions. Through expert elicitation, DIs are matched with each scenario and ranked by their causal impact, creating a unique cluster of indicators that can identify each scenario, and guide the strategic planning process.
Key Term
Definition
Driving Indicator (DI)
A Driving Indicator is a variable or factor that significantly influences the unfolding of a scenario within a framework of collective intelligence. These indicators are vital for understanding and predicting changes in specific situations or scenarios. They can be quantitative (e.g., economic growth, number of Covid deaths in a given year) or qualitative (e.g., a leader’s reputation within the international community, public confidence in institutions) and are chosen for their causal impact and relationship with the outcomes of interest.
Forecast Resolution Council
A Forecast Resolution Council refers to a group of experts or representatives assigned to agree upon and establish the interpretation and implications of Driving Indicators (or in some cases, Forecasting Variables – see below). They calibrate their understanding of each DI to ensure consistent interpretation across the team. Resolution Councils are also responsible for determining when and how an event (like a forecasted scenario) is considered to have occurred, based on agreed-upon indicators and metrics, when the Resolution Mechanism for a given forecast is complex.
Forecast Resolution Mechanism
A Forecast Resolution Mechanism is a systematic method or process used to resolve the outcomes of forecasts, typically reliant on a single, specified data source or index. A simple resolution mechanism cleanly defines the metrics or states of the future that constitute a resolution of the forecast (i.e., whether the predicted event occurred or not), as well as the procedural steps to assess the actual outcome against the forecast. In situations where the resolution mechanism is complex, a Resolution Council. An agreed-upon resolution mechanism is then used by the Forecast Resolution Council to ensure consistency and objectivity in determining the accuracy of forecasts.
Ensuring Computability: Definition of Forecastable Variables and Temporal and Spatial Boundaries
In the next stage of the Quantified Collective Intelligence framework, we engage in the critical task of transmuting Driving Indicators (DIs) into Forecastable Variables (FVs). This transformation aims to distill the essence of DIs, i.e., the core elements that significantly contribute to their impact on the given scenarios. The objective is to capture the most relevant and influential aspects of DIs, while at the same time making them amenable to systematic forecasting.
A Forecasting Variable is a key concept in the realm of decision-making and future-planning. This component can be delineated as a quantifiable metric or a definable future state that can be quantified, and therefore predicted. Examples of Forecasting Variables are diverse and context-dependent, ranging from the unemployment rate in a specific country, to changes in the technology landscape, such as the launch of a consumer-grade augmented reality (AR) device in the US market.
In order to transform these variables into actionable forecasting questions, they need a temporal context or a “forecast horizon”. A forecast horizon refers to the specific timeframe within which the prediction is expected to transpire. FVs also require spatial delineation – is the context local, national, global? Defining the boundaries of a prediction is pivotal because the same variable can lead to a myriad of distinct forecasting questions when different horizons and geographies are applied.
For instance, consider the Driving Indicator, “Widespread Penetration of Zero Emissions Vehicles.” To fully capture what’s important about this DI, you’d want to consider a range of potential Forecasting Variables, likely using SME expertise to hone in on potential tipping points in the technology, market, and regulatory environments. One specific FV might be: “Number of electric cars sold”, which you could later use to answer questions like, “When will sales to consumers of zero emissions vehicles exceed the sales of vehicles with combustion engines in China?” When a forecast horizon is applied, this FV can be utilized as the basis for various forecasting questions that help to map the landscape, such as “How many electric cars will be sold in China by December 31, 2025?,” “What will be the cumulative global sales of electric cars in the year 2025?” or “By which month in 2025 will electric car sales in China cross the 1 million mark?”
Similarly,consider the variable “Growth of the artificial intelligence market”. This could lead to forecasting questions like “What will the size of the AI market be at the end of Q2 2025?”, “What will be the year-over-year growth rate of the AI market in 2025?” or “By which year will the global AI market reach $500 billion?”
These examples highlight the critical role of the forecast horizon and spatial definition in shaping forecasting questions. Not only do they provide temporal and spatial boundaries for predictions, but they also drive the specificity and precision of the forecasted outcomes, aiding decision-makers in their strategic planning.
To accomplish the conversion of DIs to FVs, we integrate specialized knowledge from Subject Matter Experts (SMEs), together with the predictive acumen of professional forecasters. The blend of these two perspectives allows us to draw from a broad spectrum of understanding and expertise, creating a holistic representation of the DIs in the form of FVs.
A key facet of this process is reaching a consensus on the definition of the resolvable metrics that the FVs will encompass, as well as the resolution mechanisms that will be employed to track and assess these metrics. This is achieved through skilled facilitation, fostering dialogue and agreement among the experts.
Forecasting Variables, whether they’re quantifiable metrics or discernable future states, are central to the process of decision-making and strategic planning. They form the crux of any forecasting exercise, enabling the conversion of Driving Indicators (DIs) into pragmatic, forecastable entities that can be effectively analyzed and predicted.
Goal
Method
Identify Factors Relevant to DIs
Each Driving Indicator will be influenced by a number of events that could potentially occur in the world. For example, a country’s economic growth will be impacted by a wide variety of factors, such as inflation, trade relationships, consumer confidence, and geopolitical events. Defining Forecasting Variables for these factors can provide a clearer understanding of the reasons for changes in the DIs and identify the key risks and outcomes that influence the DI.
Create a Shared Understanding
Forecasts are most useful when the differences in probabilities stem from the mental models, biases, and assumptions each forecaster holds. To be able to compare predictions, forecasters must have a shared understanding of what they’re predicting. Without a shared understanding, additional variability would be introduced into the predictions due to varying interpretations of the outcome of interest. A shared understanding is accomplished by specifying metrics and resolution mechanisms for the Forecasting Variable that are clearly defined and which limit the possible range of interpretations.
Determine Relevant Forecast Horizons
Identifying appropriate forecast horizons is a critical step. An event which occurs one year from now will impact a DI differently than if it were to happen five years from now. Using the judgment of SMEs and professional forecasters, appropriate forecast horizons can be selected to ensure that the resulting probabilistic predictions are well-suited to inform our understanding of the DIs and scenarios.
Establish Appropriate Forecast Spatial Boundaries
Determining the suitable spatial boundaries for a forecast is an equally crucial step in the process. A phenomenon occurring in one geographical region may influence a DI differently than if it transpired in another. The regional economic and sociopolitical dynamics, technological advancements, and cultural aspects could all influence the unfolding of the scenario, and therefore the applicability of forecasts in the decision-making process. Leveraging the insights of Subject Matter Experts (SMEs) and professional forecasters, appropriate spatial boundaries should be defined.
Amplifying Interpretability: Designing A Concept Diagram
The creation of a Concept Diagram is a central task in the Amplifying Interpretability stage of our methodology. The objective is to graphically articulate the complex interrelationships among the system’s components and to explicitly illustrate causal pathways where they exist. The Concept Diagram, in its capacity as a graphical representation, provides a comprehensive perspective of the strategic decision-making landscape.
This diagram is constructed utilizing the data derived from previous stages, specifically the identification of Driving Indicators and the definition of Forecastable Variables. These form the critical nodes of the diagram. The associations between these nodes are meticulously delineated, establishing clear visual connectors between related elements and indicating the directionality of influence through appropriate symbology.
The process of creating this diagram enhances the depth of understanding of system dynamics. By visually representing connections between various components, it enables stakeholders to comprehend potential cascading effects instigated by changes in one part of the system. Critically, it illuminates key nodal points in the system, where strategic interventions could potentially yield substantive impacts.
Example Concept Diagram produced by a Metaculus Pro Forecaster
Revealing More: Mechanistic Mapping
The primary purpose of Mechanistic Mapping is to delve deeper into the interconnections between Driving Indicators (DIs) and Forecastable Variables (FVs). This step of the framework seeks to unpack the multifaceted mechanisms within the system being studied, and expand our understanding of the interplay among its various components. A Mechanistic Mapping exercise is not always needed, but sometimes recommended depending on the needs of the project.
This exploration begins with the process of expert elicitation, involving the input and judgment of subject matter experts. These experts are asked to identify relevant and impactful pairwise associations between the DIs and FVs. The significance of these associations is ranked, effectively establishing a hierarchy of interactions based on their perceived impact on the system’s dynamics.
Following this identification and ranking process, we engage in conditional forecasting rounds. These rounds employ the forecastable variables as conditions and evaluate how changes in these conditions affect the driving indicators and ultimately the scenarios in question. This approach further refines our understanding of the system’s dynamics and brings into focus the mechanisms that underlie the relationships between key components of the system.
The insights derived from the Mechanistic Mapping process provide decision-makers with a nuanced understanding of the system’s dynamics and the potential repercussions of various potential future events. This understanding equips them with additional context that contributes to making informed strategic decisions.
Harnessing Collective Expertise: Collaborative Forecasting with Metaculus Pro Forecasters and Subject Matter Experts
The concept of “Harnessing Collective Expertise” lies at the core of our methodological framework, leveraging the knowledge and expertise of both Metaculus Pro Forecasters and relevant Subject Matter Experts (SMEs). This approach encourages the emergence of a unique, combined forecasting power, capitalizing on the specialized insights of experts from diverse backgrounds and areas of expertise. This collaborative forecasting process bridges the gap between academic theory and practical application, offering a rich foundation of theory-based and experiential insights.
The first phase of this collaborative forecasting process employs an abbreviated implementation of the Delphi technique, a structured expert elicitation process originally developed by RAND Corporation as a systematic, interactive forecasting method. Each round of the Delphi process begins with individual forecasting, during which each participant uses their expert knowledge to make a prediction based on the provided information. This forecasting stage is accompanied by each individual documenting an explanation of the reasoning behind their prediction, encouraging transparency and enabling a deeper understanding of the diverse perspectives present.
Upon completion of the individual forecasting stage, we move on to structured small group discussions. These discussions allow the participants to compare, challenge, and refine their forecasts in light of the views presented by others. The results of these discussions are aggregated into a single collective forecast. This method not only harnesses the power of collective intelligence but also serves to balance potential individual biases, contributing to the overall robustness and reliability of the forecast. By integrating these diverse expert inputs, the collective forecasting process creates a refined, comprehensive, and nuanced forecast that can more accurately inform strategic decision-making.
Asynchronous forecasting may be undertaken during this stage of the process by Pro Forecasters, if required.
Concept
Description
Metaculus Pro Forecasters
Metaculus recruits Pro Forecasters who have demonstrated excellent forecasting ability and who have a history of clearly describing their rationales. We engage Pro Forecasters on projects where accuracy and descriptive reasoning are of the utmost importance. By working with SMEs, Pro Forecasters can employ sound forecasting practices in combination with knowledge gained and feedback received from discussions with SMEs to improve their forecasts.
Delphi Method
The Delphi method involves making individual forecasts followed by group discussion of the resulting forecasts. Initial predictions allow participants to understand where and by how much their expectation differs from that of other participants. Discussion with the group identifies the reasons for these differences, sharing missing information and differing views between participants. The process concludes with participants updating their forecasts to reflect changes in their understanding that arose from the discussion.
Aggregating Forecasts
Aggregation of forecasts is key to producing the most accurate predictions. Evidence suggests that aggregated predictions are more accurate than individual predictions on average, due to the counteraction of biases within individual predictions and inclusion of a broader base of knowledge. Aggregation unlocks the “wisdom of the crowd” and ensures more robust predictions.
Preparing for Action: Eliciting Policy Proposals
As we navigate towards the strategic application of the insights gained so far, this next phase of the process sets the groundwork for action, directing the gathered intelligence towards the pragmatic goal of influencing policy-making and strategic decision-making processes within the organization.
The first step is comprehensively mapping the available actions or policy levers. These levers represent the means by which the organization can exert influence over the Forecastable Variables, and subsequently, the Driving Indicators, and ultimately, the end-state scenarios. Each policy lever should be carefully identified, considering the unique context and capabilities of the organization, its sphere of influence, resources, and position. A list of potential actions should be enumerated, with support from both internal and external experts. These actions encapsulate the practical steps the organization can take to make progress toward their goals, protect against identified risks, or become more resilient.
Upon the delineation of potential actions, the expertise of Subject Matter Experts (SMEs) is again leveraged to assess the expected impact and utility of each action. SMEs provide their expert judgment to rank the potential actions, indicating the degree to which they believe each action will influence the desired outcomes. This step provides a preliminary view of the potential effectiveness of each action, assisting in the prioritization of strategies that have the most promising potential to yield desired results.
In this way, the process of eliciting policy proposals not only structures and organizes potential actions, but also provides a preliminary assessment of their likely effectiveness. This process aligns the decision-making process with the collective intelligence gathered, ensuring that strategic decisions are informed by expert analysis.
Grist for Decision-Making: Causal Forecasting
The process culminates with causal forecasting, where the proposed actions are evaluated in terms of their potential impact on Driving Indicators (DIs) and thus on the likely unfolding of the various scenarios. This critical phase leverages the power of forecasting, and crucially of proven, calibrated forecasters, to derive actionable insights which serve as the foundation for informed decision-making.
Causal forecasting involves producing predictions on the impact of specific actions, while meticulously controlling for potential confounding variables. Confounding variables, if not accounted for, can distort the true relationship between the implemented actions and their impact on DIs. By controlling these variables, a more accurate depiction of the causal relationships can be obtained.
Under this procedure, each proposed action is subject to a conditional forecast. This implies that the effect of each action on the DIs is measured under the condition that all other variables are held constant. The result is a clearer picture of the potential outcomes and their probabilities, enabling the organization to better understand the expected ramifications of each strategic move.
Here are two examples of causal forecasting questions within the adoption of zero-emission vehicles context:
“Assuming that government subsidies for zero-emission vehicles remain constant, how would a 10% reduction in the cost of electric vehicle (EV) battery production affect the market share of zero-emission vehicles in the U.S. by 2030?”
This question examines the causal relationship between the cost of EV battery production (a significant factor in the overall cost of zero-emission vehicles) and the adoption rate of these vehicles. In order to make it a causal forecasting question, forecasters would hold the influence of government subsidies (and potentially other variables) constant, in order to isolate the effect of battery cost reduction.
2. “If the number of EV charging stations in the U.S. were to double by 2025, what effect would this have on the percentage of new car sales that are zero-emission vehicles in 2030, assuming no significant changes in the price of gasoline?”
This question aims to forecast the impact of the availability of EV charging infrastructure on the adoption of zero-emission vehicles. It assumes that the price of gasoline, another potential influential factor, remains constant.
Both questions illustrate the concept of causal forecasting: they are designed to forecast the impact of changes in one variable (cost of battery production or number of charging stations), assuming that other potentially influential variables are held constant.
Causal forecasting serves as the engine that transforms collective intelligence and nuanced forecasting into actionable, insightful directives. This mechanism not only integrates the valuable insights gathered throughout the process but also aligns them with organizational goals, facilitating informed, strategic decision-making that is firmly grounded in expert knowledge and quantified projections. It is only made possible and effective when it is incorporated in a larger framework of quantifying collective intelligence, which ensures that the right questions are being asked, the most important actions considered, and the relevant scenarios are underpinning the entire process.
In Conclusion
The Quantified Collective Intelligence approach signifies a major advancement in integrating forecasting into strategic decision-making. It harmoniously merges diverse elements such as expert wisdom, analytical models, and illustrative diagrams to formulate a methodology that is not just robust in its predictive accuracy, but also agile in its adaptability to dynamic scenarios and transparent in its underlying mechanisms.
The strength of this approach lies in its versatility. It utilizes structured scenario development to cover a broad spectrum of possibilities, while the identification of Driving Indicators anchors these scenarios in reality. The transformation of these Indicators into Forecastable Variables establishes a concrete basis for prediction, further supported by the visual elucidation provided by Concept Diagrams and Mechanistic Mapping. The combination of expert and crowd-sourced forecasting creates a rich tapestry of insights, which, when subjected to the rigors of causal forecasting, results in highly actionable information.
In conclusion, the Quantified Collective Intelligence methodology offers a structured yet flexible framework for navigating an uncertain future. It synergistically brings together diverse elements of forecasting and scenario planning, underpinned by expert knowledge and participatory engagement. This comprehensive approach lends itself to a broad array of contexts, making it a versatile tool for organizations seeking to strategically leverage collective intelligence for decision-making in an increasingly complex and uncertain world.
Acknowledgements
Huge thanks to my teammate at Metaculus, Ryan Beck, for his contributions to the forecasting sections of this whitepaper, and to Ramsay Brown for his helpful feedback and encouragement.
Quantified Collective Intelligence: Integrating Forecasting into Decision-Making
Please note: This framework is a work-in-progress that has only been trialed a few times, and this post serves as an invitation to readers to comment—it is open to improvement!
Introduction
This post describes a methodology for the application of quantified collective intelligence (QCI) to decision-making in a policy context, leveraging the combined advantages of expert knowledge and calibrated human judgmental forecasting. The structured combination of these elements can buttress the process of decision-making and policy development, reducing bias and noise and increasing transparency and interpretability, thereby potentially leading to more robust and resilient plans and outcomes.
Components of the Quantified Collective Intelligence Framework
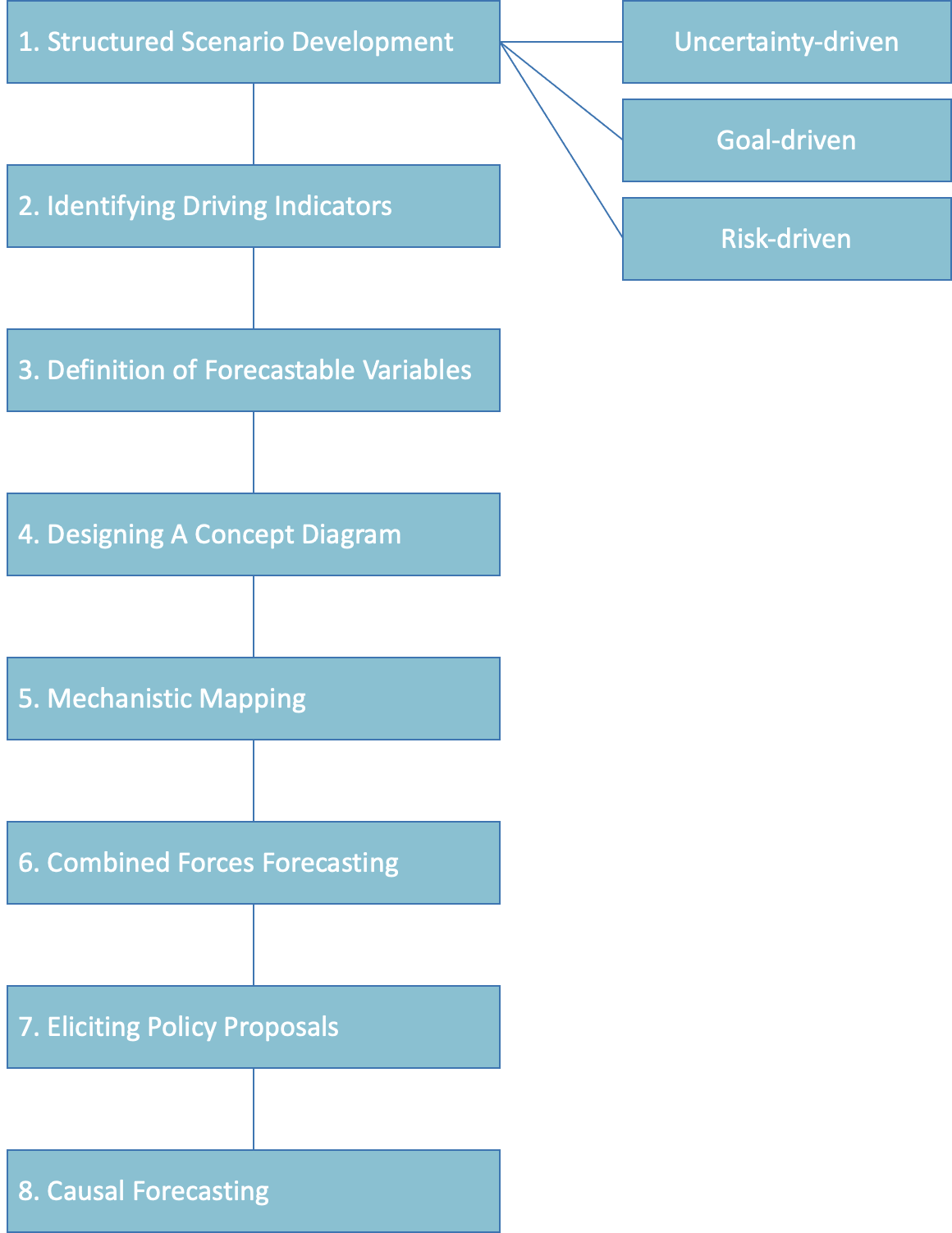
Laying the Groundwork: Structured Scenario Development
Facilitating the development of scenarios is the first step, since they will serve as a foundation for the decision-making process. The scenarios can be organized around any one of three potential pillars: Uncertainty-driven scenarios allow for versatile and flexible decision-making (utilizing a 2x2, or n x n matrix format), goal-driven scenarios are centered on a defined vision, acting as a beacon for future strategic moves, and risk-driven scenarios are tailored to anticipate and prepare for known risks, ensuring organizational resilience.
Uncertainty-driven Scenarios
Goal-driven Scenarios
Risk-driven Scenarios
Nature and Focus
Types of Uncertainty
Process & Expertise
Timeframes
Examples
Uncertainty-driven Scenarios: 2x2, n x n
These types of scenarios are designed to explore the potential impact of various uncertainties, usually across multiple dimensions, hence the use of the 2x2 or n x n matrices to represent the most important axes of uncertainty at hand. For example, a 2x2 matrix could be set up with the economy on one axis (ranging from strong to weak) and the environment on the other (ranging from stable to volatile). Four scenarios would then be generated: strong economy-stable environment, weak economy-stable environment, strong economy-volatile environment, and weak economy-volatile environment. The aim of this approach is to create a breadth of potential futures that cover a wide spectrum of possibilities, thereby preparing the organization or decision-maker for a diverse range of outcomes.
Goal-driven Scenarios: Move toward a clearly defined vision
Unlike uncertainty-driven scenarios, goal-driven scenarios start with a clear vision or end goal in mind. This approach backcasts from a desired future to the present, plotting a course that bridges the gap between the current reality and the envisioned future. Goal-driven scenarios make sense when the relevant stakeholders have already defined where they want the organization to go, and have some idea of the steps needed to get there. It’s beneficial in terms of building commitment and understanding of the goal across an organization, and is particularly useful for situations where there’s a high level of agreement about the preferred future, and the challenge lies in defining how to achieve it.
Risk-driven Scenarios: Ensure robustness against known risks
Risk-driven scenarios focus on ensuring robustness and resilience against known or identified risks. They model the impacts of specific risks and how they can be mitigated or managed. This type of scenario planning requires the identification of key risks, understanding their potential impacts, and then developing scenarios that test different response strategies. The primary objective is to stress-test strategies against these risks to ensure resilience. It provides an in-depth understanding of specific threats, assists in the preparation for potential disruptions, and allows for proactive risk management.
Quantitative Capture of Mental Models in Scenario Construction
A vital part of scenario construction lies in the understanding and translation of mental models into quantifiable entities. Mental models, representing individual or collective understanding and interpretation of how the world works, serve as the foundation upon which scenarios are built. These models help identify key variables, the relationships among them, and their potential future states, providing a basis for the narrative of the scenario. When these mental models are captured quantitatively, they become computationally tractable objects, lending themselves to precise and consistent analysis and supporting more accurate decision-making and future learning. The process of capturing mental models can be achieved through techniques such as Bayesian networks, system dynamics, or agent-based modeling, which can represent complex interdependencies and uncertainties.
By facilitating the capture of mental models into their quantified counterparts during scenario construction, we can integrate them into the QCI framework, allowing these models to evolve dynamically and be carried through each step of the overarching decision-making process.
Getting Leverage: Identifying Driving Indicators
The next stage involves Identifying Driving Indicators (DIs). These are factors that directly influence scenarios and are therefore crucial to our ability to track progress toward a given scenario or end state. A DI can be measurable or qualitative, as long as the group of experts can agree on its definition and value, allowing for its later integration into a resolution council process during the forecasting stage. Examples of quantitative DI’s might be economic growth, or the number of Covid deaths in a given year, whereas qualitative DI’s might be a leader’s reputation within the international community, or public confidence in institutions. Through expert elicitation, DIs are matched with each scenario and ranked by their causal impact, creating a unique cluster of indicators that can identify each scenario, and guide the strategic planning process.
Key Term
Definition
Driving Indicator (DI)
Forecast Resolution Council
Forecast Resolution Mechanism
Ensuring Computability: Definition of Forecastable Variables and Temporal and Spatial Boundaries
In the next stage of the Quantified Collective Intelligence framework, we engage in the critical task of transmuting Driving Indicators (DIs) into Forecastable Variables (FVs). This transformation aims to distill the essence of DIs, i.e., the core elements that significantly contribute to their impact on the given scenarios. The objective is to capture the most relevant and influential aspects of DIs, while at the same time making them amenable to systematic forecasting.
A Forecasting Variable is a key concept in the realm of decision-making and future-planning. This component can be delineated as a quantifiable metric or a definable future state that can be quantified, and therefore predicted. Examples of Forecasting Variables are diverse and context-dependent, ranging from the unemployment rate in a specific country, to changes in the technology landscape, such as the launch of a consumer-grade augmented reality (AR) device in the US market.
In order to transform these variables into actionable forecasting questions, they need a temporal context or a “forecast horizon”. A forecast horizon refers to the specific timeframe within which the prediction is expected to transpire. FVs also require spatial delineation – is the context local, national, global? Defining the boundaries of a prediction is pivotal because the same variable can lead to a myriad of distinct forecasting questions when different horizons and geographies are applied.
For instance, consider the Driving Indicator, “Widespread Penetration of Zero Emissions Vehicles.” To fully capture what’s important about this DI, you’d want to consider a range of potential Forecasting Variables, likely using SME expertise to hone in on potential tipping points in the technology, market, and regulatory environments. One specific FV might be: “Number of electric cars sold”, which you could later use to answer questions like, “When will sales to consumers of zero emissions vehicles exceed the sales of vehicles with combustion engines in China?” When a forecast horizon is applied, this FV can be utilized as the basis for various forecasting questions that help to map the landscape, such as “How many electric cars will be sold in China by December 31, 2025?,” “What will be the cumulative global sales of electric cars in the year 2025?” or “By which month in 2025 will electric car sales in China cross the 1 million mark?”
Similarly,consider the variable “Growth of the artificial intelligence market”. This could lead to forecasting questions like “What will the size of the AI market be at the end of Q2 2025?”, “What will be the year-over-year growth rate of the AI market in 2025?” or “By which year will the global AI market reach $500 billion?”
These examples highlight the critical role of the forecast horizon and spatial definition in shaping forecasting questions. Not only do they provide temporal and spatial boundaries for predictions, but they also drive the specificity and precision of the forecasted outcomes, aiding decision-makers in their strategic planning.
To accomplish the conversion of DIs to FVs, we integrate specialized knowledge from Subject Matter Experts (SMEs), together with the predictive acumen of professional forecasters. The blend of these two perspectives allows us to draw from a broad spectrum of understanding and expertise, creating a holistic representation of the DIs in the form of FVs.
A key facet of this process is reaching a consensus on the definition of the resolvable metrics that the FVs will encompass, as well as the resolution mechanisms that will be employed to track and assess these metrics. This is achieved through skilled facilitation, fostering dialogue and agreement among the experts.
Forecasting Variables, whether they’re quantifiable metrics or discernable future states, are central to the process of decision-making and strategic planning. They form the crux of any forecasting exercise, enabling the conversion of Driving Indicators (DIs) into pragmatic, forecastable entities that can be effectively analyzed and predicted.
Goal
Method
Identify Factors Relevant to DIs
Create a Shared Understanding
Determine Relevant Forecast Horizons
Establish Appropriate Forecast Spatial Boundaries
Amplifying Interpretability: Designing A Concept Diagram
The creation of a Concept Diagram is a central task in the Amplifying Interpretability stage of our methodology. The objective is to graphically articulate the complex interrelationships among the system’s components and to explicitly illustrate causal pathways where they exist. The Concept Diagram, in its capacity as a graphical representation, provides a comprehensive perspective of the strategic decision-making landscape.
This diagram is constructed utilizing the data derived from previous stages, specifically the identification of Driving Indicators and the definition of Forecastable Variables. These form the critical nodes of the diagram. The associations between these nodes are meticulously delineated, establishing clear visual connectors between related elements and indicating the directionality of influence through appropriate symbology.
The process of creating this diagram enhances the depth of understanding of system dynamics. By visually representing connections between various components, it enables stakeholders to comprehend potential cascading effects instigated by changes in one part of the system. Critically, it illuminates key nodal points in the system, where strategic interventions could potentially yield substantive impacts.
Example Concept Diagram produced by a Metaculus Pro Forecaster
Revealing More: Mechanistic Mapping
The primary purpose of Mechanistic Mapping is to delve deeper into the interconnections between Driving Indicators (DIs) and Forecastable Variables (FVs). This step of the framework seeks to unpack the multifaceted mechanisms within the system being studied, and expand our understanding of the interplay among its various components. A Mechanistic Mapping exercise is not always needed, but sometimes recommended depending on the needs of the project.
This exploration begins with the process of expert elicitation, involving the input and judgment of subject matter experts. These experts are asked to identify relevant and impactful pairwise associations between the DIs and FVs. The significance of these associations is ranked, effectively establishing a hierarchy of interactions based on their perceived impact on the system’s dynamics.
Following this identification and ranking process, we engage in conditional forecasting rounds. These rounds employ the forecastable variables as conditions and evaluate how changes in these conditions affect the driving indicators and ultimately the scenarios in question. This approach further refines our understanding of the system’s dynamics and brings into focus the mechanisms that underlie the relationships between key components of the system.
The insights derived from the Mechanistic Mapping process provide decision-makers with a nuanced understanding of the system’s dynamics and the potential repercussions of various potential future events. This understanding equips them with additional context that contributes to making informed strategic decisions.
Harnessing Collective Expertise: Collaborative Forecasting with Metaculus Pro Forecasters and Subject Matter Experts
The concept of “Harnessing Collective Expertise” lies at the core of our methodological framework, leveraging the knowledge and expertise of both Metaculus Pro Forecasters and relevant Subject Matter Experts (SMEs). This approach encourages the emergence of a unique, combined forecasting power, capitalizing on the specialized insights of experts from diverse backgrounds and areas of expertise. This collaborative forecasting process bridges the gap between academic theory and practical application, offering a rich foundation of theory-based and experiential insights.
The first phase of this collaborative forecasting process employs an abbreviated implementation of the Delphi technique, a structured expert elicitation process originally developed by RAND Corporation as a systematic, interactive forecasting method. Each round of the Delphi process begins with individual forecasting, during which each participant uses their expert knowledge to make a prediction based on the provided information. This forecasting stage is accompanied by each individual documenting an explanation of the reasoning behind their prediction, encouraging transparency and enabling a deeper understanding of the diverse perspectives present.
Upon completion of the individual forecasting stage, we move on to structured small group discussions. These discussions allow the participants to compare, challenge, and refine their forecasts in light of the views presented by others. The results of these discussions are aggregated into a single collective forecast. This method not only harnesses the power of collective intelligence but also serves to balance potential individual biases, contributing to the overall robustness and reliability of the forecast. By integrating these diverse expert inputs, the collective forecasting process creates a refined, comprehensive, and nuanced forecast that can more accurately inform strategic decision-making.
Asynchronous forecasting may be undertaken during this stage of the process by Pro Forecasters, if required.
Concept
Description
Metaculus Pro Forecasters
Delphi Method
Aggregating Forecasts
Preparing for Action: Eliciting Policy Proposals
As we navigate towards the strategic application of the insights gained so far, this next phase of the process sets the groundwork for action, directing the gathered intelligence towards the pragmatic goal of influencing policy-making and strategic decision-making processes within the organization.
The first step is comprehensively mapping the available actions or policy levers. These levers represent the means by which the organization can exert influence over the Forecastable Variables, and subsequently, the Driving Indicators, and ultimately, the end-state scenarios. Each policy lever should be carefully identified, considering the unique context and capabilities of the organization, its sphere of influence, resources, and position. A list of potential actions should be enumerated, with support from both internal and external experts. These actions encapsulate the practical steps the organization can take to make progress toward their goals, protect against identified risks, or become more resilient.
Upon the delineation of potential actions, the expertise of Subject Matter Experts (SMEs) is again leveraged to assess the expected impact and utility of each action. SMEs provide their expert judgment to rank the potential actions, indicating the degree to which they believe each action will influence the desired outcomes. This step provides a preliminary view of the potential effectiveness of each action, assisting in the prioritization of strategies that have the most promising potential to yield desired results.
In this way, the process of eliciting policy proposals not only structures and organizes potential actions, but also provides a preliminary assessment of their likely effectiveness. This process aligns the decision-making process with the collective intelligence gathered, ensuring that strategic decisions are informed by expert analysis.
Grist for Decision-Making: Causal Forecasting
The process culminates with causal forecasting, where the proposed actions are evaluated in terms of their potential impact on Driving Indicators (DIs) and thus on the likely unfolding of the various scenarios. This critical phase leverages the power of forecasting, and crucially of proven, calibrated forecasters, to derive actionable insights which serve as the foundation for informed decision-making.
Causal forecasting involves producing predictions on the impact of specific actions, while meticulously controlling for potential confounding variables. Confounding variables, if not accounted for, can distort the true relationship between the implemented actions and their impact on DIs. By controlling these variables, a more accurate depiction of the causal relationships can be obtained.
Under this procedure, each proposed action is subject to a conditional forecast. This implies that the effect of each action on the DIs is measured under the condition that all other variables are held constant. The result is a clearer picture of the potential outcomes and their probabilities, enabling the organization to better understand the expected ramifications of each strategic move.
Here are two examples of causal forecasting questions within the adoption of zero-emission vehicles context:
“Assuming that government subsidies for zero-emission vehicles remain constant, how would a 10% reduction in the cost of electric vehicle (EV) battery production affect the market share of zero-emission vehicles in the U.S. by 2030?”
This question examines the causal relationship between the cost of EV battery production (a significant factor in the overall cost of zero-emission vehicles) and the adoption rate of these vehicles. In order to make it a causal forecasting question, forecasters would hold the influence of government subsidies (and potentially other variables) constant, in order to isolate the effect of battery cost reduction.
2. “If the number of EV charging stations in the U.S. were to double by 2025, what effect would this have on the percentage of new car sales that are zero-emission vehicles in 2030, assuming no significant changes in the price of gasoline?”
This question aims to forecast the impact of the availability of EV charging infrastructure on the adoption of zero-emission vehicles. It assumes that the price of gasoline, another potential influential factor, remains constant.
Both questions illustrate the concept of causal forecasting: they are designed to forecast the impact of changes in one variable (cost of battery production or number of charging stations), assuming that other potentially influential variables are held constant.
Causal forecasting serves as the engine that transforms collective intelligence and nuanced forecasting into actionable, insightful directives. This mechanism not only integrates the valuable insights gathered throughout the process but also aligns them with organizational goals, facilitating informed, strategic decision-making that is firmly grounded in expert knowledge and quantified projections. It is only made possible and effective when it is incorporated in a larger framework of quantifying collective intelligence, which ensures that the right questions are being asked, the most important actions considered, and the relevant scenarios are underpinning the entire process.
In Conclusion
The Quantified Collective Intelligence approach signifies a major advancement in integrating forecasting into strategic decision-making. It harmoniously merges diverse elements such as expert wisdom, analytical models, and illustrative diagrams to formulate a methodology that is not just robust in its predictive accuracy, but also agile in its adaptability to dynamic scenarios and transparent in its underlying mechanisms.
The strength of this approach lies in its versatility. It utilizes structured scenario development to cover a broad spectrum of possibilities, while the identification of Driving Indicators anchors these scenarios in reality. The transformation of these Indicators into Forecastable Variables establishes a concrete basis for prediction, further supported by the visual elucidation provided by Concept Diagrams and Mechanistic Mapping. The combination of expert and crowd-sourced forecasting creates a rich tapestry of insights, which, when subjected to the rigors of causal forecasting, results in highly actionable information.
In conclusion, the Quantified Collective Intelligence methodology offers a structured yet flexible framework for navigating an uncertain future. It synergistically brings together diverse elements of forecasting and scenario planning, underpinned by expert knowledge and participatory engagement. This comprehensive approach lends itself to a broad array of contexts, making it a versatile tool for organizations seeking to strategically leverage collective intelligence for decision-making in an increasingly complex and uncertain world.
Acknowledgements
Huge thanks to my teammate at Metaculus, Ryan Beck, for his contributions to the forecasting sections of this whitepaper, and to Ramsay Brown for his helpful feedback and encouragement.